Your basket is currently empty!
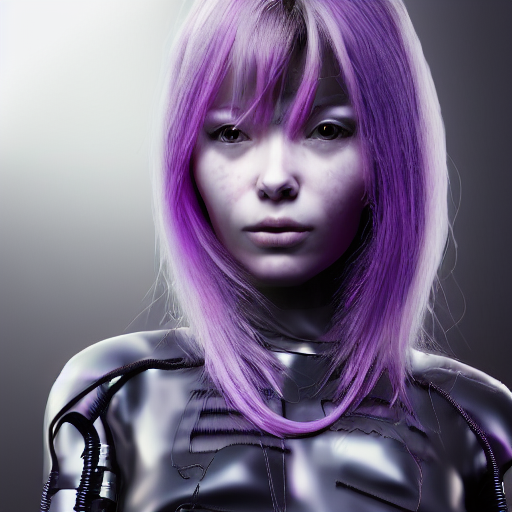
Unlocking the Power of Deep Learning: Revolutionizing Artificial Intelligence
Deep Learning (DL) is a branch of machine learning that has revolutionized the field of artificial intelligence (AI). It involves training artificial neural networks with a large number of layers to learn complex representations of data, and has enabled breakthroughs in image recognition, speech recognition, natural language processing, and other areas.
Deep Learning has its roots in the development of artificial neural networks (ANNs) in the 1940s and 1950s, but progress was slow until the 1980s, when new algorithms and computing power allowed for larger and deeper networks to be trained. In the 2010s, the advent of powerful graphics processing units (GPUs) and large datasets enabled the training of even deeper networks, leading to the current deep learning revolution.
Deep Learning is particularly suited to tasks that involve large amounts of data, such as image and speech recognition. Convolutional neural networks (CNNs) are a type of deep learning model that is commonly used for image recognition, and have achieved remarkable results on benchmarks such as the ImageNet dataset. Recurrent neural networks (RNNs) are another type of deep learning model that is well-suited to tasks involving sequences, such as natural language processing and speech recognition.
One of the key advantages of deep learning is its ability to learn representations of data that are not hand-engineered by humans. In traditional machine learning, a human expert would typically design features that the model would use to make predictions. In deep learning, the model learns these features automatically through the training process, allowing for more flexible and robust models.
Deep Learning has been used to achieve breakthroughs in a variety of applications, including self-driving cars, medical diagnosis, and drug discovery. It has also been used to develop creative applications such as style transfer, where the style of one image is applied to another image, and generative models, which can create new images, music, and other forms of art.
Despite its successes, deep learning still faces many challenges. One of the biggest challenges is the need for large amounts of labeled data to train the models. This can be a bottleneck in applications where obtaining labeled data is difficult or expensive. Another challenge is the interpretability of deep learning models, which can be difficult to understand and debug due to their complexity.
In conclusion, Deep Learning is a powerful technique for training artificial neural networks with a large number of layers to learn complex representations of data. It has enabled breakthroughs in a variety of applications and has the potential to transform many industries. While it still faces many challenges, the rapid progress in the field suggests that deep learning will continue to have a significant impact on the future of AI.
Leave a Reply
You must be logged in to post a comment.